The terms “related” and “correlated” often surface in discussions spanning from casual conversations to academic research, yet their meanings and implications are frequently misunderstood or used interchangeably. At their core, these concepts explore the connections between variables, objects, or phenomena, each with its unique set of characteristics and applications. The distinction between them is subtle but significant, reflecting the depth and nature of the relationship being examined.
The difference between related and correlated lies in the nature and measurement of the connection between two or more entities. While “related” refers to a general connection or association that may not be quantifiable, “correlated” implies a statistical relationship where changes in one variable are associated with changes in another, often measured by a correlation coefficient. This distinction is crucial for accurate interpretation and analysis in various fields.
Understanding the difference enhances our ability to interpret data, draw conclusions, and make decisions based on relationships. It empowers researchers, analysts, and even everyday individuals to distinguish between simple associations and statistically significant correlations, paving the way for informed conclusions and actions across diverse domains.
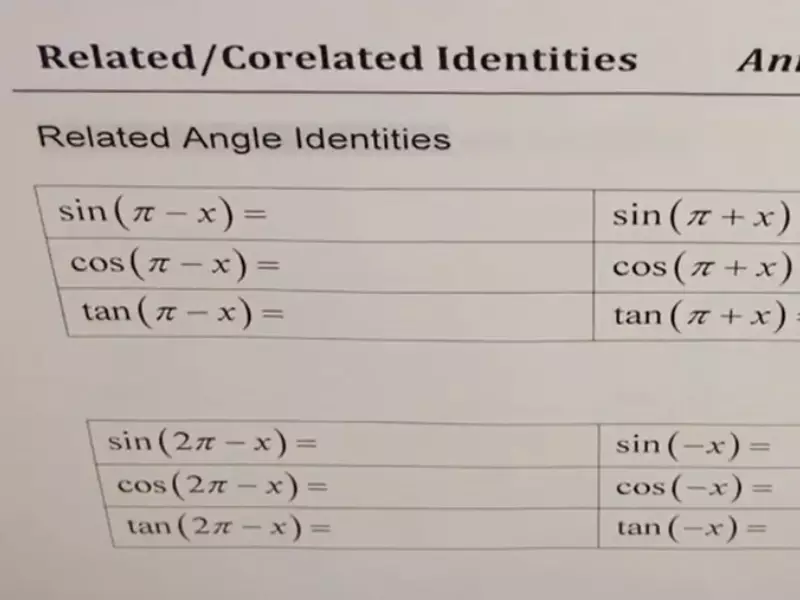
Core Concepts
What is Relation
Definition
A relation represents a connection or association between two or more entities. This connection can be based on various factors such as functionality, dependence, or association within different contexts. In essence, when entities share a common attribute or can be grouped based on a specific criterion, they are considered related.
Types of Relationships
Relationships can be categorized into several types, including:
- Functional Relationships: Where the relationship is based on a function or role. For example, a teacher and a student are related through the educational process.
- Causal Relationships: Involving cause and effect, where one entity’s action directly affects another.
- Comparative Relationships: Entities are related through comparison, such as similarities or differences.
- Sequential Relationships: Defined by a sequence or order, like steps in a process.
Examples in Different Contexts
- In mathematics, numbers can be related through equations or functions.
- In biology, species are related based on evolutionary pathways.
- In sociology, individuals are related through social networks or kinship.
What is Correlation
Definition
Correlation is a statistical measure that describes the extent to which two or more variables fluctuate together. A higher correlation implies a stronger relationship where, as one variable changes, the other variable tends to change in a predictable manner.
Types of Correlations
Correlations are mainly of two types:
- Positive Correlation: Both variables move in the same direction.
- Negative Correlation: Variables move in opposite directions.
Correlation Coefficients
The correlation coefficient is a numerical measure that quantifies the degree of correlation. Values range from -1 to 1, where:
- 1 indicates a perfect positive correlation.
- 0 indicates no correlation.
- -1 indicates a perfect negative correlation.
Comparison Criteria
Basis of Comparison
Nature
The nature of relation is often conceptual, focusing on the inherent or defined connections between entities. Correlation, by contrast, is empirical, emphasizing observable and measurable associations.
Measurement
Measurement of relation is qualitative, assessing the presence or type of connection. Correlation is quantitative, using statistical methods to calculate the strength of the relationship.
Fields of Application
Relations are applied broadly across disciplines such as mathematics, philosophy, and linguistics. Correlation is primarily used in statistics, research, and sciences to understand and predict patterns.
Relation vs Correlation
Direct Relationship vs Statistical Correlation
A direct relationship implies a clear and defined connection between entities, while statistical correlation may not imply a direct cause-and-effect relationship but indicates a pattern or trend between variables.
Qualitative Aspects vs Quantitative Analysis
Relations often involve qualitative aspects, understanding the nature of connections, whereas correlation involves quantitative analysis, focusing on the measurement of these connections.
Significance in Research
Role in Statistical Analysis
Predictive Modeling
Correlation is crucial in predictive modeling to identify variables that can predict outcomes effectively.
Hypothesis Testing
Both relation and correlation are vital in hypothesis testing, helping researchers to confirm or refute their hypotheses based on the strength and type of connections.
Application in Various Fields
Science and Medicine
In science and medicine, understanding genetic relationships or the correlation between lifestyle factors and health outcomes is essential for disease prevention and treatment.
Economics and Finance
In economics and finance, correlation helps in portfolio management, identifying how different investments move in relation to each other.
Psychology and Sociology
In psychology and sociology, exploring the relationships and correlations between social behaviors and psychological processes is key to understanding human actions and societal trends.
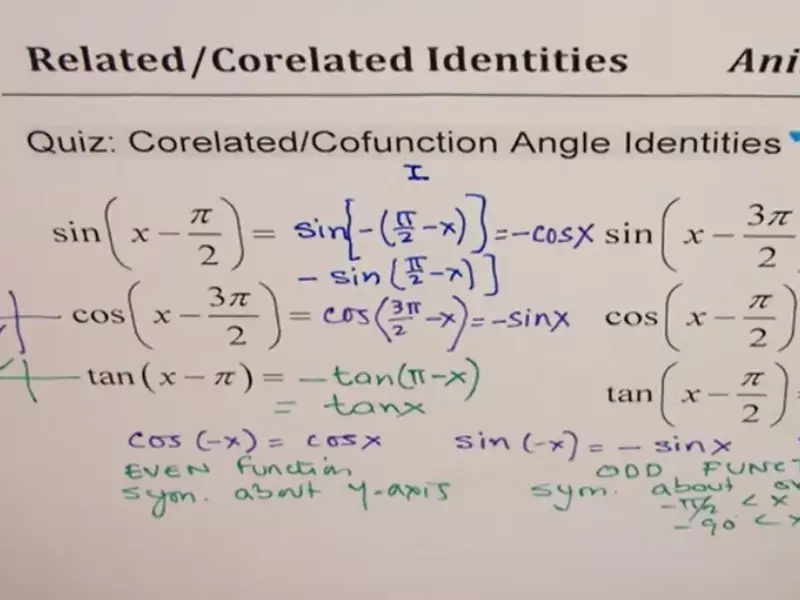
Misinterpretations
Correlation Does Not Imply Causation
One of the most crucial concepts in statistical analysis is that correlation does not imply causation. This means just because two variables move together does not mean that one causes the other. This misunderstanding can lead to incorrect assumptions and decisions.
Common Misunderstandings
Misinterpretations often arise when people see a relationship between two variables and jump to conclusions about cause and effect. For instance, a study might find a correlation between ice cream sales and shark attacks. While it may appear that ice cream sales lead to shark attacks, both are actually related to a third factor, which is the increase in temperature during summer months.
Examples of Spurious Correlations
Spurious correlations are relationships that are statistically significant but not causally related. An example could be the number of films Nicolas Cage appeared in a year correlating with the number of swimming pool drownings. These correlations are coincidental and highlight the importance of not taking statistical data at face value without deeper analysis.
Analyzing Relationships
Challenges in Identifying True Relationships
Identifying true relationships between variables requires careful analysis and consideration of all possible factors. Challenges include:
- Distinguishing between correlation and causation
- The presence of lurking variables
- Bias and confounding variables
Tools and Techniques for Accurate Analysis
Several tools and techniques are essential for accurate analysis, including:
- Regression analysis to understand the relationship between variables
- Stratification to control for confounding variables
- Path analysis to explore direct and indirect relationships
Practical Examples
Real-World Applications
Case Studies in Healthcare
In healthcare, understanding the difference between correlation and causation can impact treatment and policy decisions. For example, a study might find a correlation between a specific lifestyle and reduced risk of a disease. Researchers must determine if the lifestyle directly influences disease risk or if other factors are at play.
Market Research Analysis
Market research utilizes correlation to predict consumer behavior. By analyzing purchasing patterns and customer feedback, companies can identify trends and factors that influence buying decisions. However, it’s vital to recognize that these correlations may not indicate causation.
Environmental Studies
Environmental scientists use correlation studies to identify potential risks and protect ecosystems. For instance, a correlation between pollution levels and biodiversity loss requires careful examination to determine if pollution is the direct cause or if other factors contribute to the observed pattern.
Impact on Decision Making
Business Strategy
In business, understanding the relationship between variables is key to strategic planning. Companies use correlation analysis to forecast demand, optimize operations, and develop marketing strategies. However, misinterpreting correlations can lead to ineffective or harmful strategies.
Public Policy
Public policy decisions often rely on statistical data. Policies on education, health, and environmental protection can be influenced by correlations between various factors and outcomes. Policymakers must discern between correlation and causation to implement effective and beneficial policies.
Frequently Asked Questions
What does “related” mean in a general sense?
“Related” refers to a connection or association between two or more entities. This relationship can be based on various factors such as causality, similarity, or proximity. Unlike correlation, which is quantifiable, being related is a broader concept that may not always be measured statistically.
How is correlation different from causation?
Correlation indicates a statistical relationship between two variables where changes in one are associated with changes in the other. However, it does not imply that one causes the other. Causation, on the other hand, means that one event is the direct result of another. Establishing causation requires more rigorous testing beyond finding a correlation.
Why is understanding the difference between related and correlated important?
Understanding the difference is crucial for accurate data interpretation and analysis. It helps in distinguishing between mere associations and statistically significant relationships, which is essential for making informed decisions in research, policy-making, business strategy, and everyday problem-solving.
Can two variables be related without being correlated?
Yes, two variables can be related without being statistically correlated. This situation occurs when there is a connection or association between the variables that is not linear or does not fit a pattern that can be measured by a correlation coefficient. Examples include non-linear relationships or connections based on qualitative characteristics.
Conclusion
Grasping the distinction between related and correlated is more than an academic exercise; it is a fundamental aspect of critical thinking and data literacy. This understanding equips individuals with the ability to discern the nature of relationships between variables, enhancing the quality of analysis, research findings, and decision-making processes across various sectors.
As we continue to navigate an increasingly data-driven world, the ability to accurately interpret connections and correlations becomes indispensable. The nuanced understanding of these concepts not only aids in avoiding common pitfalls like assuming causation from correlation but also fosters a deeper appreciation for the complexity of the relationships that define our world.